AI is hot. The word is heard regularly in every (virtual) boardroom and gone are the days when you first had to write the term in full to make it clear what you are talking about. Moreover, there are already thousands of ready-made algorithms that you can use, sometimes for free and sometimes for a fee. Nothing stands in the way of companies getting started, you might say. Yet far from all organisations are achieving success with AI. This is because the quality of the data and the context in which the data is collected determine the quality of AI outcomes. ‘Garbage in is garbage out’. So you will have to find a way to keep control over the data you use.
Companies often think that a big data quality project is the only way to gain control over the data. Such a project is time-consuming and expensive, which is why many do not embark on it. Nevertheless, it is important to understand in advance not only the quality of data (accuracy, completeness, timeliness), but also things like definitions used, the context in which data are generated and the way data are recorded (e.g. is a five- or seven-point scale used for a particular measurement; is the temperature recorded in Celsius or Fahrenheit?). Because if different sources use different definitions or the data is collected in a different context, you have a huge pile of apples and pears that you cannot compare.
Business rules engine selects the appropriate data
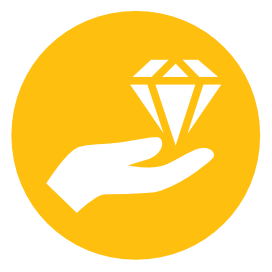
It is therefore important to properly embed AI in your organisation and create clarity about the quality of data before using it in all kinds of analyses. A relatively unknown method to do that is applying business rules. Business rules are ideal for saying in clear, understandable language how business operations should go. By doing so, you also achieve great quality and insight into the data that support those business operations. This is the quality you need to apply AI algorithms successfully.
For every data requirement, you set up a business rule. You also assign characteristics to all the data sources you use: in what context was the data collected? What do we mean by a particular term? What measurement method was used to capture a value? And so on. Once you unleash an AI algorithm on the dataset, a business rules engine will make sure that your analysis only includes data that complies with the business rules you set up. This way, you avoid having to rig up a very large data quality project before you can start using AI. You simply use all the data that is available and the business rules engine determines which data is and is not included in a specific analysis on the basis of the rules you have drawn up yourself.
Unambiguous understanding of what certain data mean
With this method, you also guarantee that everyone who is going to do something with the data understands what the data mean. After all, this is laid down in the business rules and these are written in clear language that everyone can understand. In many organisations, there are no unambiguous data definitions. One department understands a term like ‘outage’ or ‘customer’ differently from another, so you can never analyse data from different systems in context. However, if you overlay those systems with a business rules engine, you force different departments or users to talk to each other about the meaning of data in their systems. Does the data meet the set conditions? Which data can and cannot be included in an analysis?
Business rules engine enforces that different departments start talking to each other
Taking away data noise

We illustrate with an example. A medical researcher wants to investigate situations that trigger palpitations in patients. He has access to as many as 30 different databases with heart rate data from anonymised patients: databases from ICU departments, databases with data from holter analyses (a box that allows patients to walk around freely while being monitored) and also data that people collected themselves with a heart rate monitor. For this researcher, it is very important to know in what context and with what type of device the data was collected. In business rules, he can define exactly which rules data must comply with to be included in a specific analysis. He does not have to check all data sources beforehand; he can limit himself to recording the properties of the data in the database. If those properties meet the business rules he has set up, then those data are included in the analysis and otherwise they are disregarded. In this way, he significantly reduces the time he spends on ‘data stuff’. This is because he no longer has to check each field separately, but can suffice with determining the meaning of the data in a given row or column and setting rules. For example: if a field in a row is empty, that entire row is not included in the analysis. Or: the value of this field must not be higher than x or lower than y. This way, it prevents, for example, typing errors, such as a comma in the wrong place, from disrupting the analysis.
Keep the overview by using human language
he great value of this approach is that the people who want to do something with the data can make their own requirements. If you perform this administration in an analytics environment like R, the users of the data quickly lose the overview. Because in R, data is in a form suitable for the algorithm. This is a form that data scientists may understand, but users do not. However, users are the domain experts who have to indicate which data should or should not be included in an analysis. There is then a high risk that due to tuning problems, the wrong data will still be included, e.g. heart rate data from smart watches while the research goal is to investigate palpitations in patients at rest.
This approach has great added value because people who want to do something can set their own data requirements
Start small and ‘grow as you go
Another great advantage of a business rules approach is that you can start small, with just a few data types for which you set up rules. You then don’t have to make sky-high investments beforehand, but use a ‘grow as you go’ scenario. This is attractive, because every organisation has processes that everyone involved knows can be organised more efficiently or effectively, but nothing is done about them because, although the solution is hidden ‘somewhere in the data’, there is no ready-made solution that allows you to find the answer. Think, for instance, of complex spreadsheets that are only really understood by a few people, schedules of different departments that are not properly aligned or decisions that are still made time and again based on gut feelings, when in essence there is enough data available to make a more rational decision. In these situations, you can start very small with a business rules approach, with perhaps only two or three types of data that you analyse in conjunction. Chances are that the insights you gain from this will inspire new analysis ideas, which in turn will require new data sources. In this way, it will grow naturally, without immediately requiring large investments and freeing up your people completely for this project.
In short, want to get governance on AI right from the start and reduce the complexity around data administration? Then consider a business rules approach.
This article was also published in AG Connect, 11-2020.
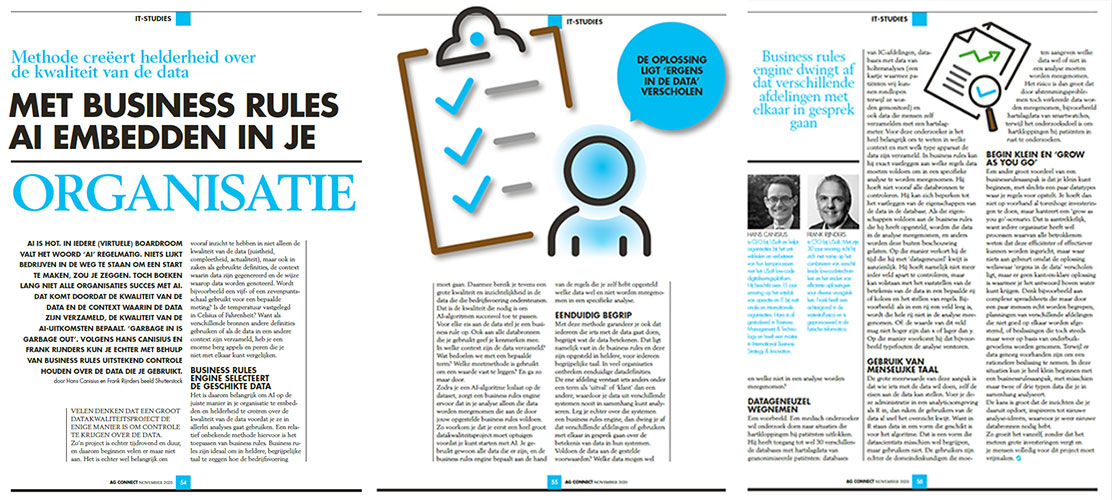
About
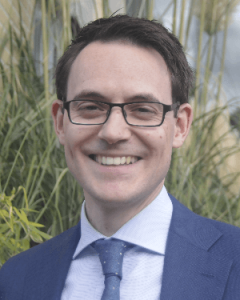
Hans Canisius
CEO at USoft since February 2020, helping organisations develop and improve their core processes with the USoft low-code digitisation platform. He has 15 years of experience at the intersection of operations and IT at national and international organisations. Hans holds a degree in Business Management & Technology and a Masters in International Business Strategy & Innovation.

Frank Rijnders
CTO at USoft. With his 30 years of experience, he focuses mainly on combining various low-code technologies and finding efficient solutions for various issues. Frank has a background in solid state physics and a PhD in physical computer science.